Mobile Personalization with AI: Beyond Recommendations
In the ever-evolving landscape of mobile technology, personalization stands as a cornerstone for delivering compelling user experiences. From tailored recommendations to customized interfaces, the quest for relevance and engagement drives the integration of artificial intelligence (AI) into mobile applications. While recommendations have long been the focal point of personalization efforts, the potential of AI extends far beyond mere product suggestions.
This article delves into the realm of mobile personalization with AI, exploring the transformative power of advanced algorithms and data analytics. Beyond the traditional paradigm of recommendations, we examine how AI enables a new frontier of customization, adaptation, and proactive engagement. By leveraging AI-driven insights, mobile apps can not only anticipate user needs but also dynamically adjust content and interactions in real-time.
Current State of Mobile Personalization
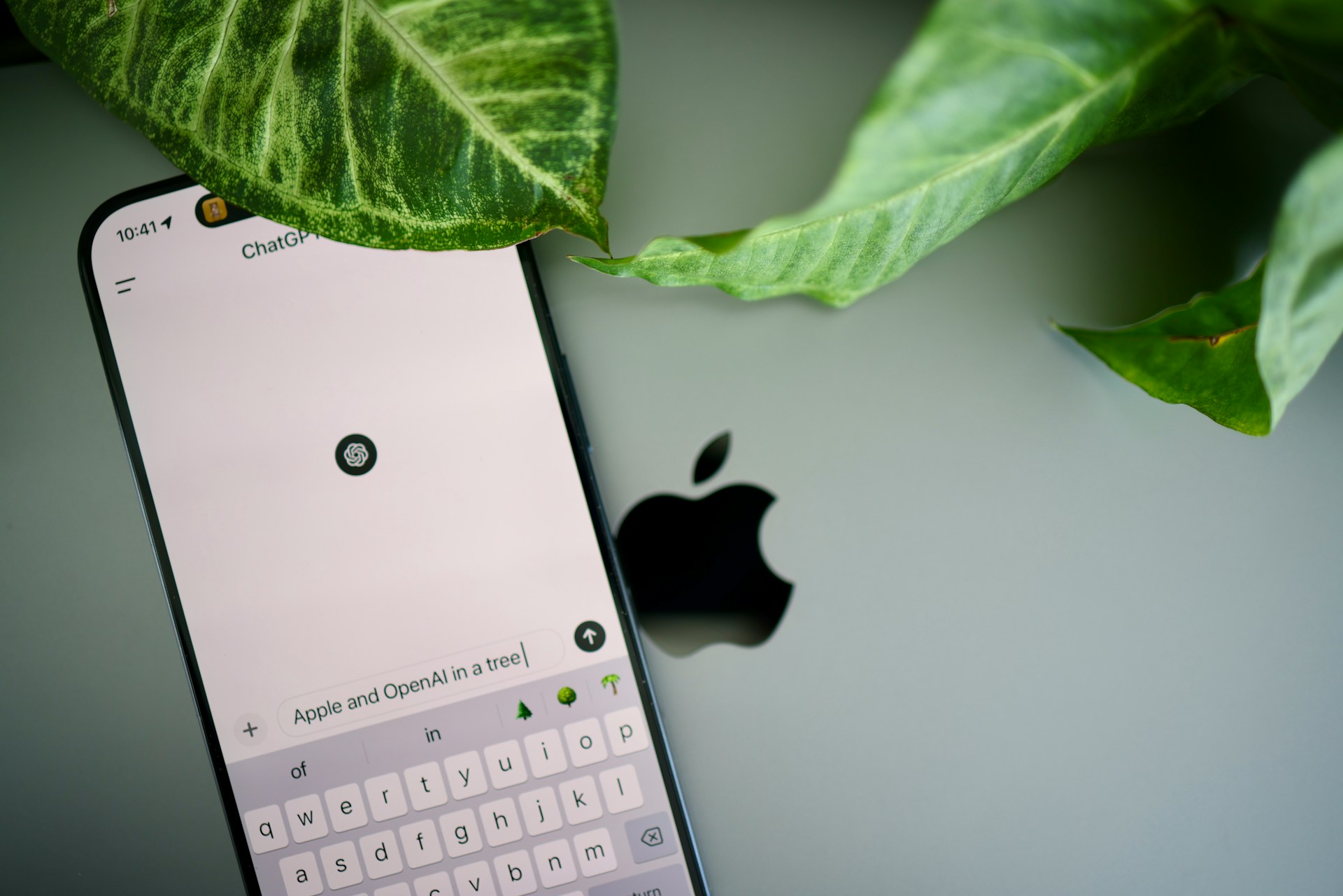
In today's digital landscape, mobile personalization has become ubiquitous, with recommendation systems serving as the backbone of many user experiences. These systems analyze user behavior, preferences, and historical data to generate product suggestions, content recommendations, and targeted advertisements. While undeniably valuable, the current state of mobile personalization faces several challenges and limitations.
Overview of Existing Recommendation Systems
- Algorithmic-based recommendations: Most recommendation systems rely on algorithms such as collaborative filtering, content-based filtering, and hybrid approaches to generate personalized suggestions.
- Application across industries: Recommendation systems are widely used across various sectors, including e-commerce, media streaming, social networking, and news aggregation.
- User feedback mechanisms: Many platforms incorporate user feedback mechanisms to refine recommendations over time, improving their accuracy and relevance.
Limitations and Challenges Faced
- Limited scope of personalization: Traditional recommendation systems often focus solely on product recommendations, overlooking other aspects of user experience such as interface customization and proactive engagement.
- Cold-start problem: New users or items with limited data pose a challenge for recommendation systems, leading to less accurate and relevant suggestions.
- Over-reliance on historical data: Recommendation algorithms may suffer from biases and stereotypes present in historical data, potentially leading to discriminatory or unbalanced recommendations.
- Privacy concerns: The collection and analysis of user data raise privacy concerns, prompting regulatory scrutiny and user distrust.
Beyond Recommendations: Expanding the Scope with AI
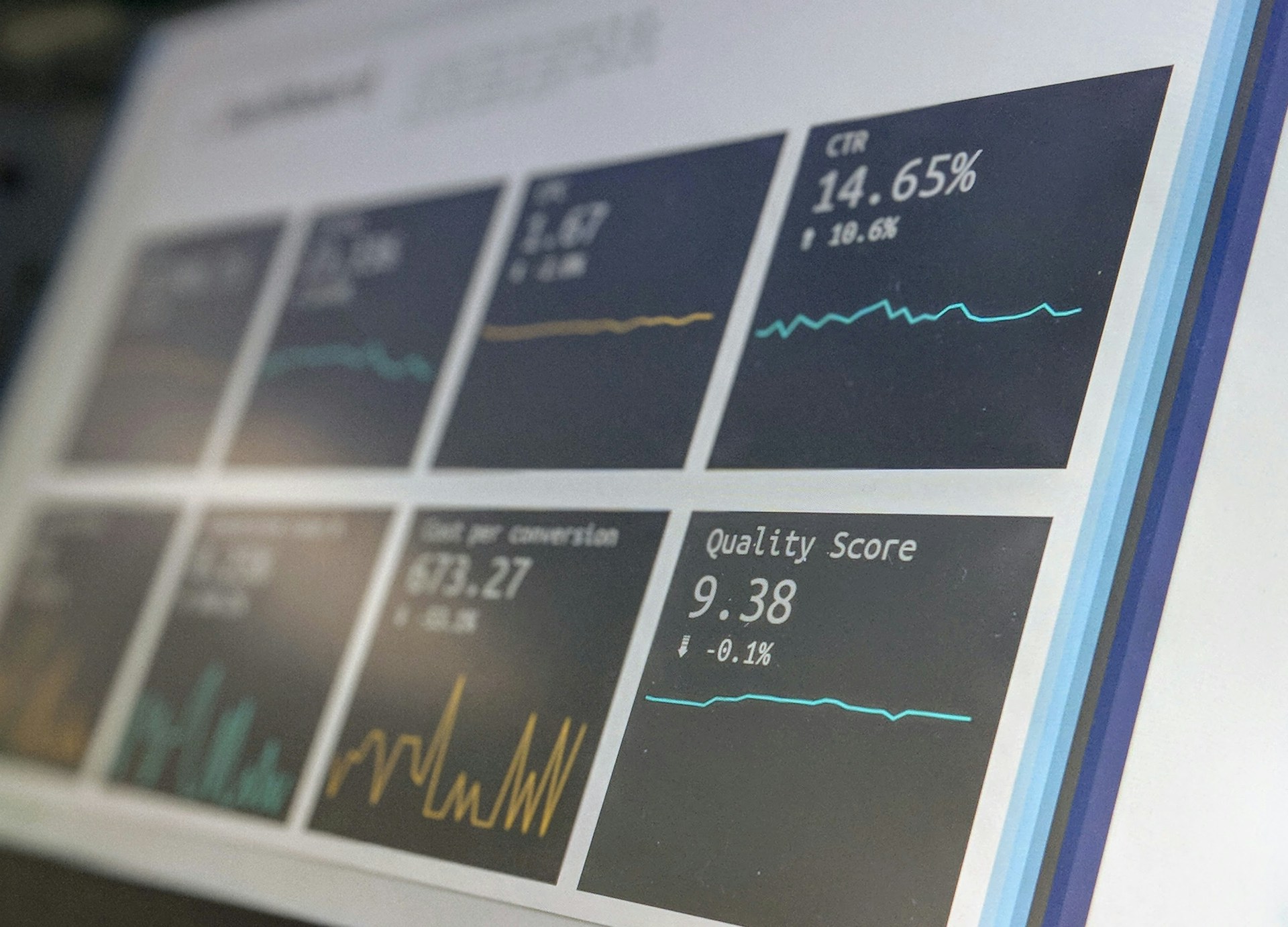
While recommendations have long been the cornerstone of mobile personalization, the capabilities of artificial intelligence (AI) extend far beyond suggesting products or content. With AI-driven techniques, mobile applications can offer customized user experiences that adapt to individual preferences, behaviors, and contexts. This section explores how AI enables a broader spectrum of personalization beyond traditional recommendations.
Customized User Experiences Beyond Product Recommendations
- Interface customization: AI algorithms can dynamically adjust the layout, design elements, and navigation paths of mobile interfaces based on user preferences and usage patterns.
- Content personalization: Beyond recommending products or articles, AI can tailor content such as news, entertainment, or educational materials to match users' interests, language proficiency, and reading habits.
- Adaptive interactions: AI-powered chatbots and virtual assistants can engage users in natural language conversations, offering personalized assistance, recommendations, and support.
Adaptive Interfaces and Content Personalization
- Contextual awareness: AI algorithms can analyze contextual signals such as location, time of day, device type, and user activity to deliver timely and relevant content and features.
- Dynamic content delivery: By continuously learning from user interactions and feedback, AI systems can dynamically adjust content recommendations, promotions, and notifications in real-time.
- Multimodal experiences: AI enables the creation of immersive and interactive experiences by integrating voice commands, gesture recognition, and augmented reality (AR) technologies into mobile applications.
Predictive Analytics for Proactive Personalization
- Anticipatory computing: AI algorithms can predict user needs and preferences before they explicitly express them, enabling proactive personalization and preemptive actions.
- Behavior-based segmentation: By clustering users based on their behavioral patterns and engagement metrics, AI systems can target specific user segments with tailored experiences and incentives.
- Lifetime value optimization: AI-driven analytics can forecast the long-term value of individual users and prioritize personalized interventions to maximize user retention, loyalty, and lifetime value.
By leveraging AI for personalized user experiences that go beyond mere recommendations, mobile applications can foster deeper engagement, loyalty, and satisfaction among their users. However, realizing the full potential of AI-driven personalization requires a concerted effort to address privacy concerns, ethical considerations, and algorithmic biases.
Leveraging AI for Enhanced User Engagement
In the pursuit of optimizing user engagement and retention, AI emerges as a powerful ally, offering insights and capabilities to dynamically adapt to user behavior and preferences. This section delves into how AI can be harnessed to elevate user engagement within mobile applications, driving meaningful interactions and fostering long-term relationships.
Real-time Behavioral Analysis
- Continuous learning: AI algorithms analyze user interactions in real-time, identifying patterns, preferences, and trends to inform personalized recommendations and content delivery.
- Predictive modeling: By extrapolating from historical data, AI can anticipate future user actions and tailor the user experience accordingly, optimizing engagement and conversion rates.
- Adaptive user interfaces: AI-driven interfaces dynamically adjust based on user behavior, simplifying navigation, highlighting relevant features, and removing friction points to enhance user satisfaction.
Contextual Awareness and Dynamic Content Delivery
- Contextual relevance: AI systems leverage contextual cues such as location, time, device type, and user intent to deliver hyper-targeted content, promotions, and recommendations.
- Personalized notifications: AI-powered push notifications and alerts are strategically timed and tailored to individual preferences, increasing the likelihood of user interaction and conversion.
- Multichannel engagement: AI facilitates seamless integration across multiple channels and touchpoints, ensuring a cohesive user experience across web, mobile, social media, and messaging platforms.
Personalized Notifications and Alerts
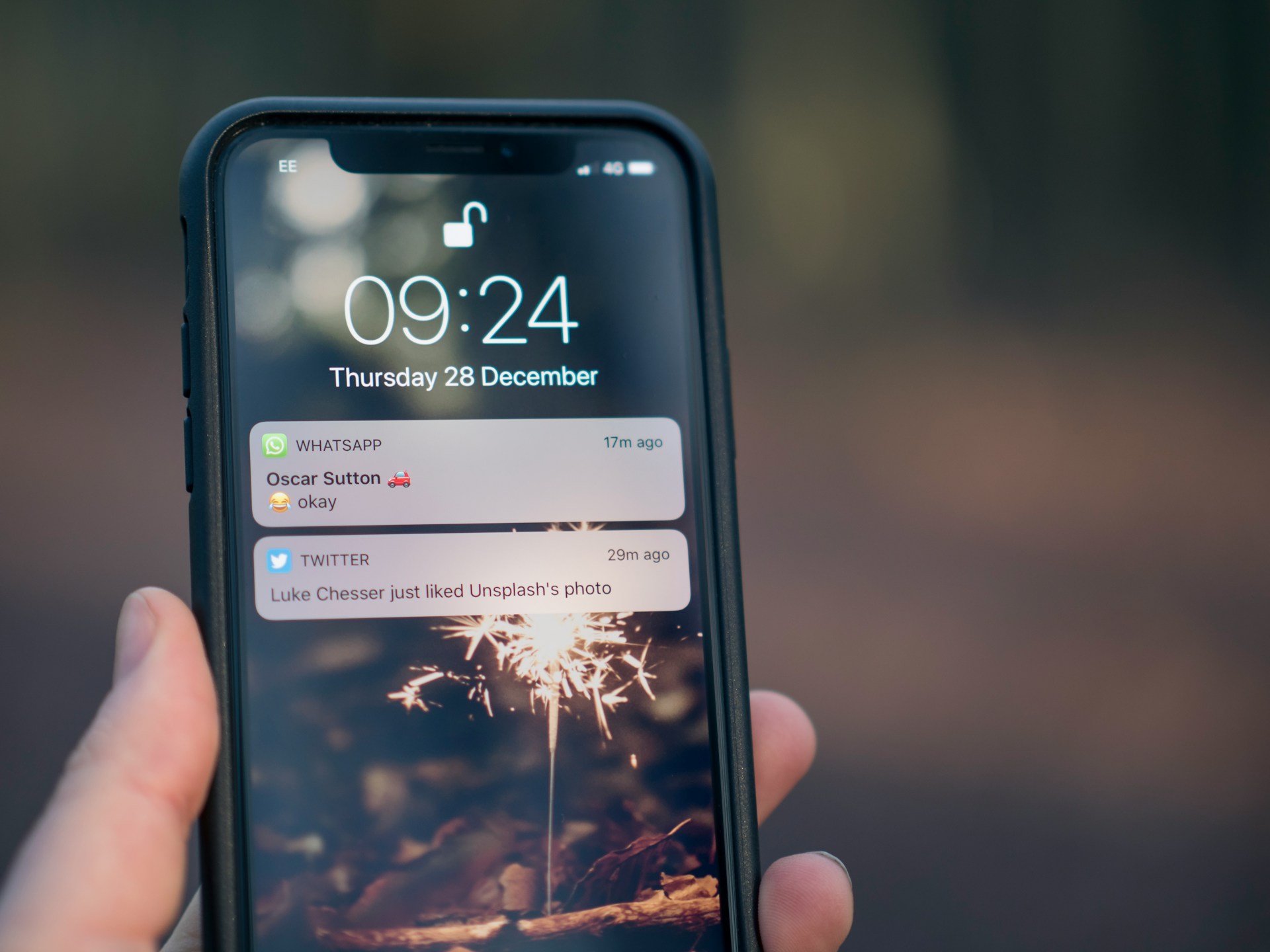
- Behavioral triggers: AI identifies key user behaviors and events that signal readiness for specific actions or interventions, enabling personalized notifications and alerts to be triggered at the right moment.
- A/B testing and optimization: AI-powered experimentation enables iterative refinement of notification strategies, identifying the most effective messaging, timing, and frequency for maximizing user engagement.
- Performance monitoring: AI analytics track the effectiveness of notifications in driving user engagement metrics such as click-through rates, conversion rates, and retention rates, enabling data-driven decision-making and continuous improvement.
By embracing AI-driven strategies for user engagement, mobile applications can cultivate deeper connections with their audience, fostering loyalty, advocacy, and lifetime value. However, achieving optimal results requires a relentless focus on efficiency, effectiveness, and user-centricity, guided by data-driven insights and a commitment to delivering exceptional user experiences.
Conclusion
By embracing a culture of transparency and providing users with clear explanations of how their data is used, mobile applications can build trust and confidence among their user base. Moreover, by implementing robust data security measures and proactively addressing algorithmic biases, companies can mitigate the risks of data misuse and discrimination.
If you are interested in how AI and AR in mobile apps are transforming user experiences, check out our in-depth articles covering these innovations across education and other industries.